Phys.org  October 30, 2023
The existing deep-learning methods for holographic imaging often depend solely on the specific condition based on the given data distributions. One critical problem is how to guarantee the alignment between any given downstream tasks and pretrained models. Researchers in China analyzed the physical mechanism of image degradation caused by turbulence and proposed a swin transformer-based method, termed train-with-coherence-swin (TWC-Swin) transformer, which used spatial coherence (SC) as an adaptable physical prior information to precisely align image restoration tasks in the arbitrary turbulent scene. They designed light-processing system (LPR) which enabled manipulation of SC and simulation of any turbulence. Qualitative and quantitative evaluations demonstrated that the TWC-Swin method presented superiority over traditional convolution frameworks and realized image restoration under various turbulences, which suggested its robustness, powerful generalization capabilities, and adaptability to unknown environments. According to the researchers the significance of physical prior information in the optical intersection and provides an effective solution for model-to-tasks alignment schemes, which will help to unlock the full potential of deep learning for all-weather optical imaging across terrestrial, marine, and aerial domains… read more. Open Access TECHNICAL ARTICLEÂ
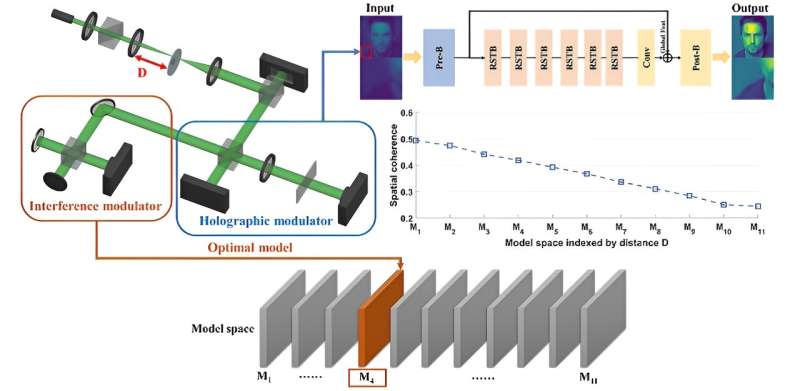
Leveraging spatial coherence as a physical prior to guide the training of a deep neural network… Credit: Advanced Photonics, Vol. 5, Issue 6, 066003, October 2023Â