MIT News March 30, 2023
While neural networks are used for classification tasks across domains, a long-standing open problem in machine learning is determining whether neural networks trained using standard procedures are consistent for classification, i.e., whether such models minimize the probability of misclassification for arbitrary data distributions. A team of researchers in the US (MIT, UC San Diego) identified, constructed and analyzed infinitely wide networks that were also infinitely deep. Using the recent connection between infinitely wide neural networks and neural tangent kernels, they provided explicit activation functions that could be used to construct networks that achieve consistency. They created a taxonomy of infinitely wide and deep networks and showed that these models implemented one of three well-known classifiers depending on the activation function used: 1) 1-nearest neighbor (model predictions are given by the label of the nearest training example); 2) majority vote (model predictions are given by the label of the class with the greatest representation in the training set); or 3) singular kernel classifiers (a set of classifiers containing those that achieve consistency). According to the researchers their results highlight the benefit of using deep networks for classification tasks, in contrast to regression tasks, where excessive depth is harmful… read more. TECHNICAL ARTICLEÂ
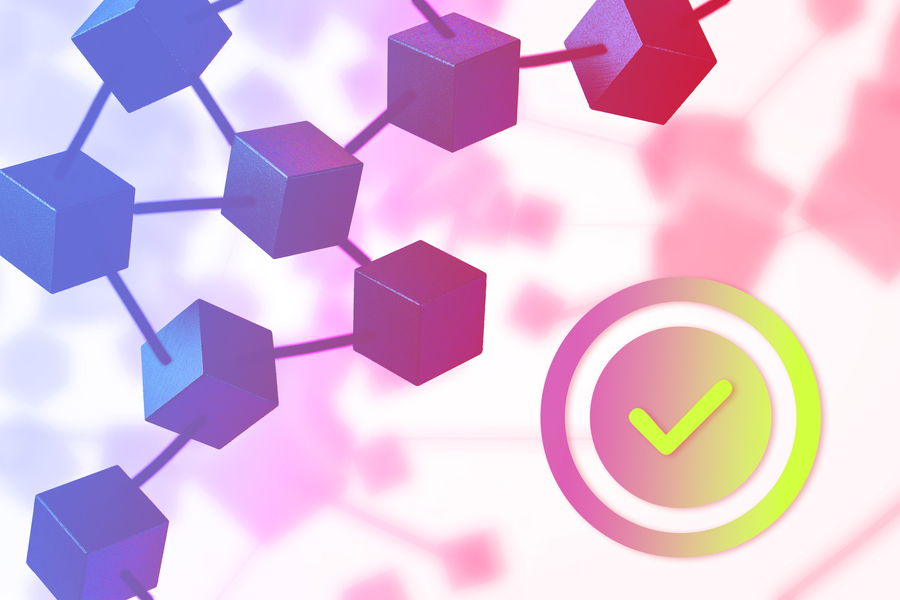
MIT researchers have found that neural networks can be designed so they minimize the probability of misclassifying a data input.
Credits: Image: MIT