MIT News August 22, 2023
Optical neural networks (ONNs) have recently emerged to process deep neural networks (DNN) tasks with high clock rates, parallelism, and low-loss data transmission. However, existing challenges for ONNs are high energy consumption due to their low electro-optic conversion efficiency, low compute density due to large device footprints and channel crosstalk, and long latency due to the lack of inline nonlinearity. An international team of researchers (USA – MIT, UCLA, industry, Germany) experimentally demonstrated a spatial-temporal-multiplexed ONN system that simultaneously overcomes all these challenges. They exploited neuron encoding with volume-manufactured micrometre-scale vertical-cavity surface-emitting laser (VCSEL) arrays that exhibited efficient electro-optic conversion and compact footprint. Homodyne photoelectric multiplication allowed matrix operations at the quantum-noise limit and detection-based optical nonlinearity with instantaneous response. With three-dimensional neural connectivity, their system could reach an energy efficiency of 7 femtojoules per operation (OP) with a compute density of 6 teraOP mm−2 s−1, representing 100-fold and 20-fold improvements, respectively, over state-of-the-art digital processors. According to the researchers near-term development could improve these metrics by two more orders of magnitude. The optoelectronic processor opens new avenues to accelerate machine learning tasks from data centres to decentralized device… read more. TECHNICAL ARTICLE
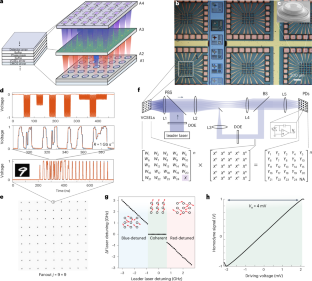
Experimental scheme of VCSEL-ONN. Credit: Nature Photonics, July 17, 2023Â