MIT News September 27, 2023
Researchers at MIT have introduced a new family of physics-inspired generative models termed PFGM++ that unifies diffusion models and Poisson Flow Generative Models (PFGM). The models produced generative trajectories for N dimensional data by embedding paths in N+D dimensional space while still controlling the progression with a simple scalar norm of the D additional variables. The new model reduced to PFGM when D=1 and to diffusion models when D→∞. The flexibility of choosing D allowed them to trade off robustness against rigidity as increasing D resulted in more concentrated coupling between the data and the additional variable norms. They dispensed with the biased large batch field targets used in PFGM and instead provided an unbiased perturbation-based objective like diffusion models. To explore different choices of D, they provided a direct alignment method for transferring well-tuned hyperparameters from diffusion models (D→∞) to any finite D values. Their experiments showed that models with finite D could be superior to previous state-of-the-art diffusion models. They demonstrated that models with smaller D exhibit improved robustness against modeling errors. They provided a link to the Code https://github.com/Newbeeer/pfgmpp… read more. Open Access TECHNICAL ARTICLEÂ
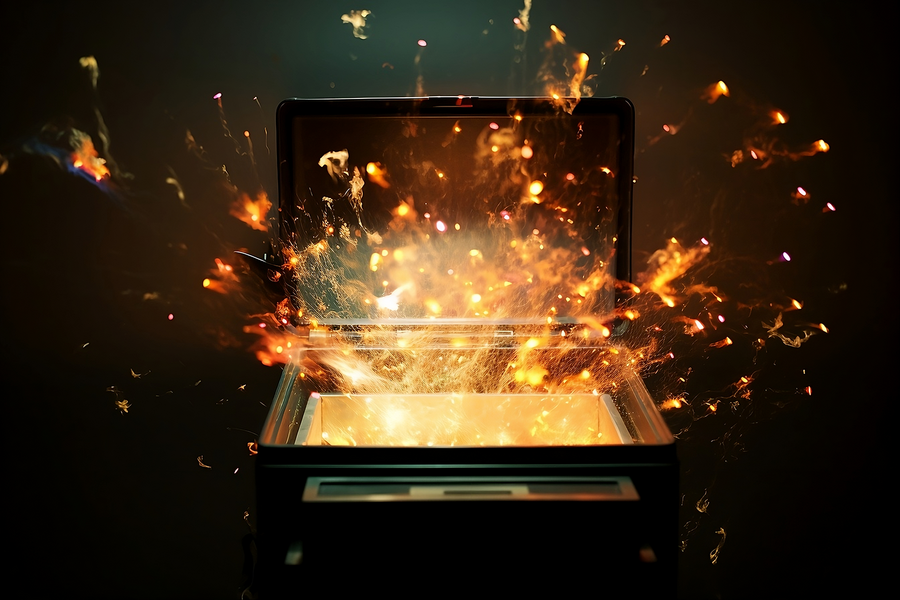
…a new family of physics-inspired generative models termed PFGM++ that unifies diffusion models and Poisson Flow Generative Models (PFGM) for better pattern recognition. Credits: Alex Shipps/MIT CSAIL via Midjourney